The Data Crunch In AI: Strategies For Sustainability
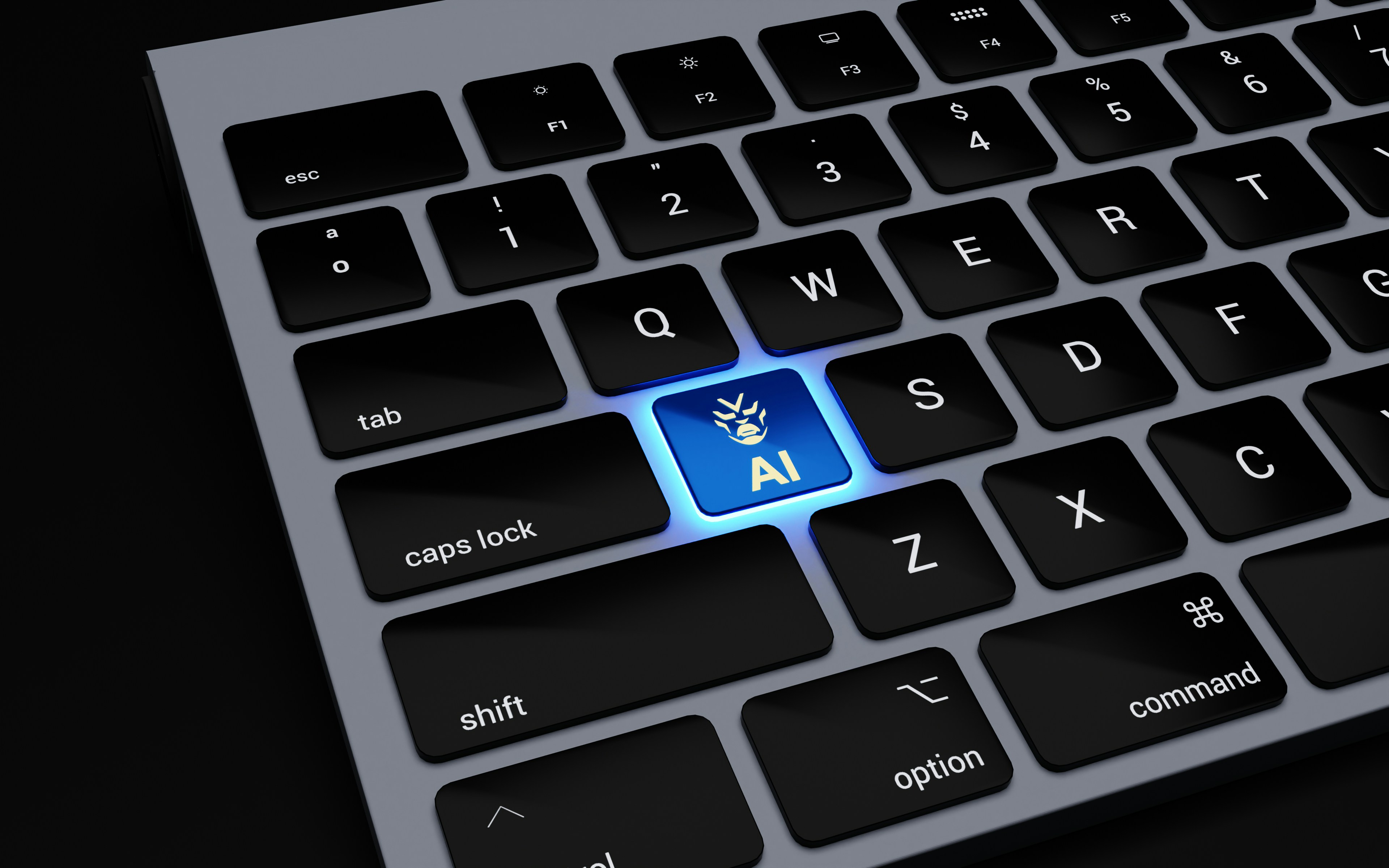
Exploring solutions to the imminent exhaustion of internet data for AI training.
As the artificial intelligence (AI) industry continues to expand, a critical challenge is emerging: the potential exhaustion of the internet's data. AI systems, particularly those that rely on machine learning, consume vast amounts of data to improve their performance. However, experts warn that this resource is finite and could soon be depleted, posing a significant threat to the future development of AI technologies.
Current Situation
AI models, especially those based on machine learning and deep learning, require extensive datasets to train their algorithms and refine their accuracy. The internet has been a rich source of this data, but with the rapid pace of AI advancements, this resource is being consumed at an unprecedented rate. Some industry analysts predict that within a few years, AI firms will exhaust most of the readily available data on the internet, leading to a critical shortage that could hinder further progress.
Synthetic Data Generation
One promising solution to this looming data scarcity is the generation of synthetic data. Synthetic data is artificially created and designed to replicate real-world scenarios. This method provides a virtually limitless supply of training material for AI models, ensuring that the development of AI technologies can continue unabated. Synthetic data can be tailored to specific needs, allowing researchers to generate datasets that are highly relevant to their particular applications.
The use of synthetic data is already gaining traction in the AI community. For example, in the field of autonomous vehicles, synthetic data is used to simulate various driving conditions and scenarios that are difficult to capture in real life. This approach not only supplements the existing data but also enhances the robustness of AI models by exposing them to a wider range of situations.
Efficient AI Algorithms
Another crucial strategy involves developing more efficient AI algorithms. By creating models that require less data to achieve high levels of accuracy, the reliance on vast amounts of data can be reduced. Efficient algorithms can optimize the use of available data, ensuring that AI systems continue to perform well even as data becomes scarcer.
Researchers are focusing on techniques such as transfer learning and federated learning to improve data efficiency. Transfer learning allows models to leverage knowledge from previously trained tasks, reducing the need for large datasets. Federated learning enables multiple AI systems to collaborate and learn from decentralized data sources, further conserving data and enhancing model performance.
Implications for Various Industries
The potential data shortage has far-reaching implications for various sectors, including healthcare, finance, and autonomous vehicles. In healthcare, AI systems are used for diagnostics and personalized medicine, relying heavily on vast amounts of patient data. A shortage could hinder advancements in these critical areas. In finance, AI is employed for risk assessment and fraud detection, where large datasets are essential for accuracy. Similarly, the development of autonomous vehicles depends on extensive data for safety and navigation, making the impending shortage a significant concern.
Collaborative Efforts and Innovation
Addressing the data crunch will require a collaborative effort between AI firms, researchers, and policymakers. Data sharing agreements and partnerships can help mitigate the shortage by pooling resources and making data more widely available. Additionally, increased funding for research into synthetic data and efficient algorithms will be crucial in developing sustainable solutions.
Policymakers also have a vital role to play in fostering an environment conducive to innovation. By creating regulations that encourage data sharing while protecting privacy, they can support the continued growth of the AI industry. Incentives for research and development in synthetic data generation and algorithm efficiency can further drive progress.
Conclusion
The impending data shortage presents a significant challenge for the AI industry, but proactive measures can ensure continued growth and innovation. By embracing synthetic data generation and developing more efficient algorithms, AI firms can mitigate the effects of data scarcity. Collaboration between industry, researchers, and policymakers will be essential in addressing this issue and securing a sustainable future for AI technologies. With the right strategies in place, the AI industry can continue to thrive despite the looming data crunch.
Author: Brett Hurll
From Chip War To Cloud War: The Next Frontier In Global Tech Competition
The global chip war, characterized by intense competition among nations and corporations for supremacy in semiconductor ... Read more
The High Stakes Of Tech Regulation: Security Risks And Market Dynamics
The influence of tech giants in the global economy continues to grow, raising crucial questions about how to balance sec... Read more
The Tyranny Of Instagram Interiors: Why It's Time To Break Free From Algorithm-Driven Aesthetics
Instagram has become a dominant force in shaping interior design trends, offering a seemingly endless stream of inspirat... Read more
Google Abandons Four-Year Effort To Remove Cookies From Chrome Browser
After four years of dedicated effort, Google has decided to abandon its plan to remove third-party cookies from its Chro... Read more
LinkedIn Embraces AI And Gamification To Drive User Engagement And Revenue
In an effort to tackle slowing revenue growth and enhance user engagement, LinkedIn is turning to artificial intelligenc... Read more
OpenAI And Broadcom Collaborate On New AI Chip To Boost Computational Power
OpenAI and Broadcom are reportedly in discussions to co-develop a new AI chip. This strategic partnership aims to enhanc... Read more