DeepMind Explores Inner Workings Of AI
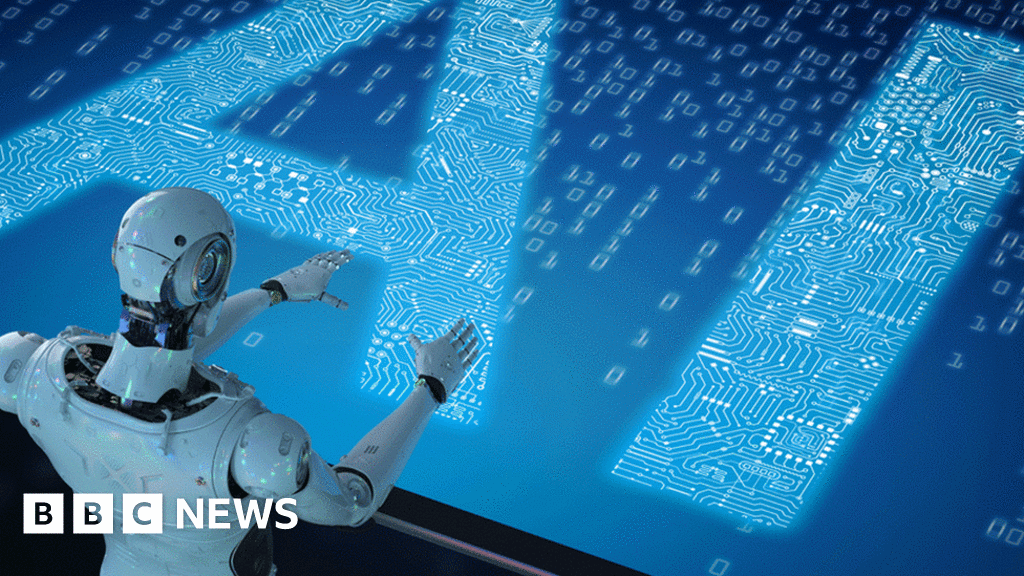
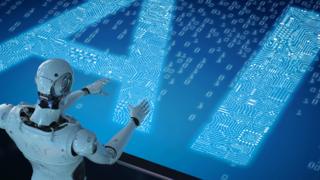
As with the human brain, the neural networks that power artificial intelligence systems are not easy to understand.
DeepMind, the Alphabet-owned AI firm famous for teaching an AI system to play Go, is attempting to work out how such systems make decisions.
By knowing how AI works, it hopes to build smarter systems.
But researchers acknowledged that the more complex the system, the harder it might be for humans to understand.
The fact that the programmers who build AI systems do not entirely know why the algorithms that power it make the decisions they do, is one of the biggest issues with the technology.
It makes some wary of it and leads others to conclude that it may result in out-of-control machines.
Just as with a human brain, neural networks rely on layers of thousands or millions of tiny connections between neurons, clusters of mathematical computations that act in the same way as the neurons in the brain.
These individual neurons combine in complex and often counter-intuitive ways to solve a wide range of challenging tasks.
"This complexity grants neural networks their power but also earns them their reputation as confusing and opaque black boxes," wrote the researchers in their paper.
According to the research, a neural network designed to recognise pictures of cats will have two different classifications of neurons working in it - interpretable neurons that respond to images of cats and confusing neurons, where it is unclear what they are responding to.
To evaluate the relative importance of these two types of neurons, the researchers deleted some to see what effect it would have on network performance.
They found that neurons that had no obvious preference for images of cats over pictures of any other animal, play as big a role in the learning process as those clearly responding just to images of cats.
They also discovered that networks built on neurons that generalise, rather than simply remembering images they had been previously shown, are more robust.
"Understanding how networks change... will help us to build new networks which memorise less and generalise more," the researchers said in a blog.
"We hope to better understand the inner workings of neural networks, and critically, to use this understanding to build more intelligent and general systems," they concluded.
However, they acknowledged that humans may still not entirely understand AI.
DeepMind research scientist Ari Morcos told the BBC: "As systems become more advanced we will definitely have to develop new techniques to understand them."
From Chip War To Cloud War: The Next Frontier In Global Tech Competition
The global chip war, characterized by intense competition among nations and corporations for supremacy in semiconductor ... Read more
The High Stakes Of Tech Regulation: Security Risks And Market Dynamics
The influence of tech giants in the global economy continues to grow, raising crucial questions about how to balance sec... Read more
The Tyranny Of Instagram Interiors: Why It's Time To Break Free From Algorithm-Driven Aesthetics
Instagram has become a dominant force in shaping interior design trends, offering a seemingly endless stream of inspirat... Read more
The Data Crunch In AI: Strategies For Sustainability
Exploring solutions to the imminent exhaustion of internet data for AI training.As the artificial intelligence (AI) indu... Read more
Google Abandons Four-Year Effort To Remove Cookies From Chrome Browser
After four years of dedicated effort, Google has decided to abandon its plan to remove third-party cookies from its Chro... Read more
LinkedIn Embraces AI And Gamification To Drive User Engagement And Revenue
In an effort to tackle slowing revenue growth and enhance user engagement, LinkedIn is turning to artificial intelligenc... Read more