AI's Rising Tide Lifts All Chips As AMD Instinct, Cloudy Silicon Vie For A Slice Of Nvidia's Pie
Nvidia dominated the AI arena in 2024, with shipments of its Hopper GPUs more than tripling to over two million among its 12 largest customers, according to estimates from Omdia.
But while Nvidia remains an AI infrastructure titan, it's facing stiffer competition than ever from rival AMD. Among earlier adopters of its Instinct MI300 series GPUs, AMD is quickly gaining share.
Omdia estimates that Microsoft purchased approximately 581,000 GPUs in 2024, the largest of any cloud or hyperscale customer in the world. Of those, one in six was built by AMD.
At Meta – by far the most enthusiastic adopter of the barely year-old accelerators, according to Omdia's findings – AMD accounted for 43 percent of GPU shipments at 173,000 versus Nvidia's 224,000. Meanwhile, at Oracle, AMD accounted for 23 percent of the database giant's 163,000 GPU shipments.
Despite growing share among key customers like Microsoft and Meta, AMD's share of the broader GPU market remains comparatively small next to Nvidia.
Omdia's estimates tracked MI300X shipments across four vendors – Microsoft, Meta, Oracle, and GPU bit barn TensorWave – which totaled 327,000.
AMD's ramp is no less notable as its MI300-series accelerators have only been on the market for a year now. Prior to that, AMD's GPUs were predominantly used in more traditional high-performance computing applications like Oak Ridge National Laboratory's (ORNL) 1.35 exaFLOPS Frontier supercomputer.
"They managed to prove the effectiveness of the GPUs through the HPC scene last year, and I think that helped," Vladimir Galabov, research director for cloud and datacenter at Omdia, told The Register. "I do think there was a thirst for an Nvidia alternative."
Why AMD?
How much of this thirst is driven by limited supply of Nvidia hardware is hard to say, but at least on paper, AMD's MI300X accelerators offered a number of advantages. Introduced a year ago, the MI300X claimed 1.3x higher floating point performance for AI workloads, as well as 60 percent higher memory bandwidth and 2.4x higher capacity than the venerable H100.
The latter two points make the part particularly attractive for inference workloads, the performance of which are more often dictated by how much and how fast your memory is rather than how many FLOPS the GPU can throw around.
Generally speaking, most AI models today are trained at 16-bit precision, which means that in order to run them, you need approximately 2 GB of vRAM for every one billion parameters. With 192 GB of HBM3 per GPU, a single server boasts 1.5 TB of vRAM. This means that large models, like Meta's Llama 3.1 405B frontier model, can run on a single node. A similarly equipped H100 node, on the other hand, lacks the memory necessary to run the model at full resolution. The 141 GB H200 doesn't suffer from this same limitation, but capacity isn't the MI300X's only party trick.
The MI300X boasts 5.3 TBps of memory bandwidth, versus 3.3 TBps on the H100 and 4.8 TBps for the 141 GB H200. Together, this means that the MI300X should in theory be able to serve larger models faster than Nvidia's Hopper GPUs.
Even with Nvidia's Blackwell, which is only just beginning to reach customers, pulling ahead on performance and memory bandwidth, AMD's new MI325X still holds a capacity advantage at 256 GB per GPU. Its more powerful MI355X slated for release late next year will push this to 288 GB.
As such, it's no surprise that Microsoft and Meta, both of which are deploying large frontier models measuring hundreds of billions or even trillions of parameters in size, have gravitated to AMD's accelerators.
This, Galabov notes, has been reflected in AMD's guidance, which has consistently inched upward quarter after quarter. As of Q3, AMD now expects Instinct to drive $5 billion in revenues in fiscal 2024.
Going into the New Year, Galabov believes that AMD has an opportunity to gain even more share. "AMD executes well. It communicates well with clients, and it's good at talking about its strengths and its weaknesses transparently," he said.
One potential driver is the emergence of GPU bit barns, like CoreWeave, which are deploying tens of thousands of accelerators a year. "Some of these are going to purposely try to build a business model around an Nvidia alternative," Galabov said, pointing to TensorWave as one such example.
Custom silicon hits its stride
It's not just AMD chipping away at Nvidia's empire. At the same time cloud and hyperscalers are buying up massive quantities of GPUs, many are deploying custom AI silicon of their own.
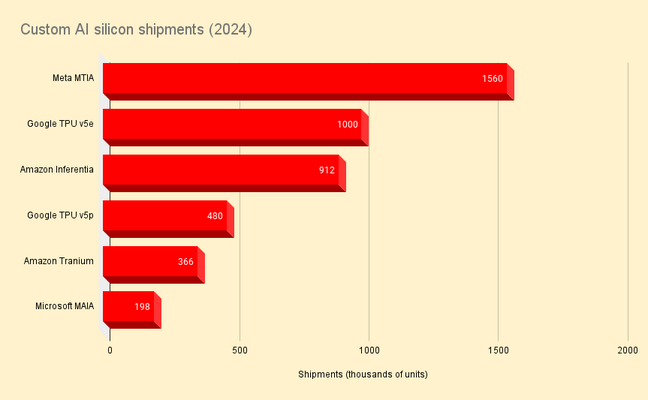
Cloud providers deployed massive quantities of custom AI silicon in 2024, but it's important to remember not all of these parts are designed for GenAI. Credit Omdia – click to enlarge
Omdia estimates that shipments of Meta's custom MTIA accelerators, which we looked at in more detail earlier this year, crested 1.5 million in 2024, while Amazon placed orders for 900,000 Inferentia chips.
Whether or not this poses a challenge to Nvidia depends pretty heavily on the workload. That's because these parts are designed to run more traditional machine learning tasks like the recommender systems used to match ads to users and products to buyers.
While Inferentia and MTIA may not have been designed with LLMs in mind, Google's TPUs certainly were and have been used to train many of the search giant's language models, including both its proprietary Gemini and open Gemma models.
Best Omdia can figure, Google placed orders for about a million TPU v5e and 480,000 TPU v5p accelerators this year.
In addition to Inferentia, AWS also has its Trainium chips, which despite their name have been retuned for both training and inference workloads. In 2024, Omdia figures Amazon ordered about 366,000 of these parts. This aligns with its plans for Project Rainier, which will provide model builder Anthropic with "hundreds of thousands" of its Trainium2 accelerators in 2025.
Finally there's Microsoft's MAIA parts, which were first teased shortly before AMD debuted the MI300X. Similar to Trainium, these parts are tuned for both inference and training, something Microsoft obviously does a fair bit of as OpenAI's main hardware partner and a model builder in its own right. Omdia believes Microsoft ordered roughly 198,000 of these parts in 2024.
- Million GPU clusters, gigawatts of power – the scale of AI defies logic
- Humanoid robots coming soon, initially under remote control
- Boffins trick AI model into giving up its secrets
- Just how deep is Nvidia's CUDA moat really?
The AI market is bigger than hardware
Nvidia's monumental revenue gains over the past two years have understandably shone a spotlight on the infrastructure behind AI, but it's only one piece of a much larger puzzle.
Omdia expects Nvidia to struggle over the next year to grow its share of the AI server market as AMD, Intel, and the cloud service providers push alternative hardware and services.
"If we've learned anything from Intel, once you've reached 90-plus percent share, it's impossible to continue to grow. People will immediately look for an alternative," Galabov said.
However, instead of fighting for share in an increasingly competitive market, Galabov suspects that Nvidia will instead focus on expanding the total addressable market by making the technology more accessible.
The introduction of Nvidia Inference Microservices (NIMs), containerized models designed to function like puzzle pieces for building complex AI systems, are just one example of this pivot.
"It's the Steve Jobs strategy. What made the smartphone successful is the App Store. Because it makes the technology easy to consume," Galabov said of NIMs. "It's the same with AI; make an app store and people will download the app and they'll use it."
Having said that, Nvidia remains grounded in hardware. Cloud providers, hyperscalers, and GPU bit barns are already announcing massive clusters based on Nvidia's powerful new Blackwell accelerators, which pull well ahead of anything AMD or Intel have to offer today, at least in terms of performance.
Meanwhile, Nvidia has accelerated its product roadmap to support a yearly cadence of new chips to maintain its lead. It seems that while Nvidia will continue to face stiff competition from its rivals it's at no risk of losing its crown any time soon. ®
From Chip War To Cloud War: The Next Frontier In Global Tech Competition
The global chip war, characterized by intense competition among nations and corporations for supremacy in semiconductor ... Read more
The High Stakes Of Tech Regulation: Security Risks And Market Dynamics
The influence of tech giants in the global economy continues to grow, raising crucial questions about how to balance sec... Read more
The Tyranny Of Instagram Interiors: Why It's Time To Break Free From Algorithm-Driven Aesthetics
Instagram has become a dominant force in shaping interior design trends, offering a seemingly endless stream of inspirat... Read more
The Data Crunch In AI: Strategies For Sustainability
Exploring solutions to the imminent exhaustion of internet data for AI training.As the artificial intelligence (AI) indu... Read more
Google Abandons Four-Year Effort To Remove Cookies From Chrome Browser
After four years of dedicated effort, Google has decided to abandon its plan to remove third-party cookies from its Chro... Read more
LinkedIn Embraces AI And Gamification To Drive User Engagement And Revenue
In an effort to tackle slowing revenue growth and enhance user engagement, LinkedIn is turning to artificial intelligenc... Read more