AI-Powered Lab Assistants: How DeepMind And BioNTech Are Changing The Face Of Scientific Research
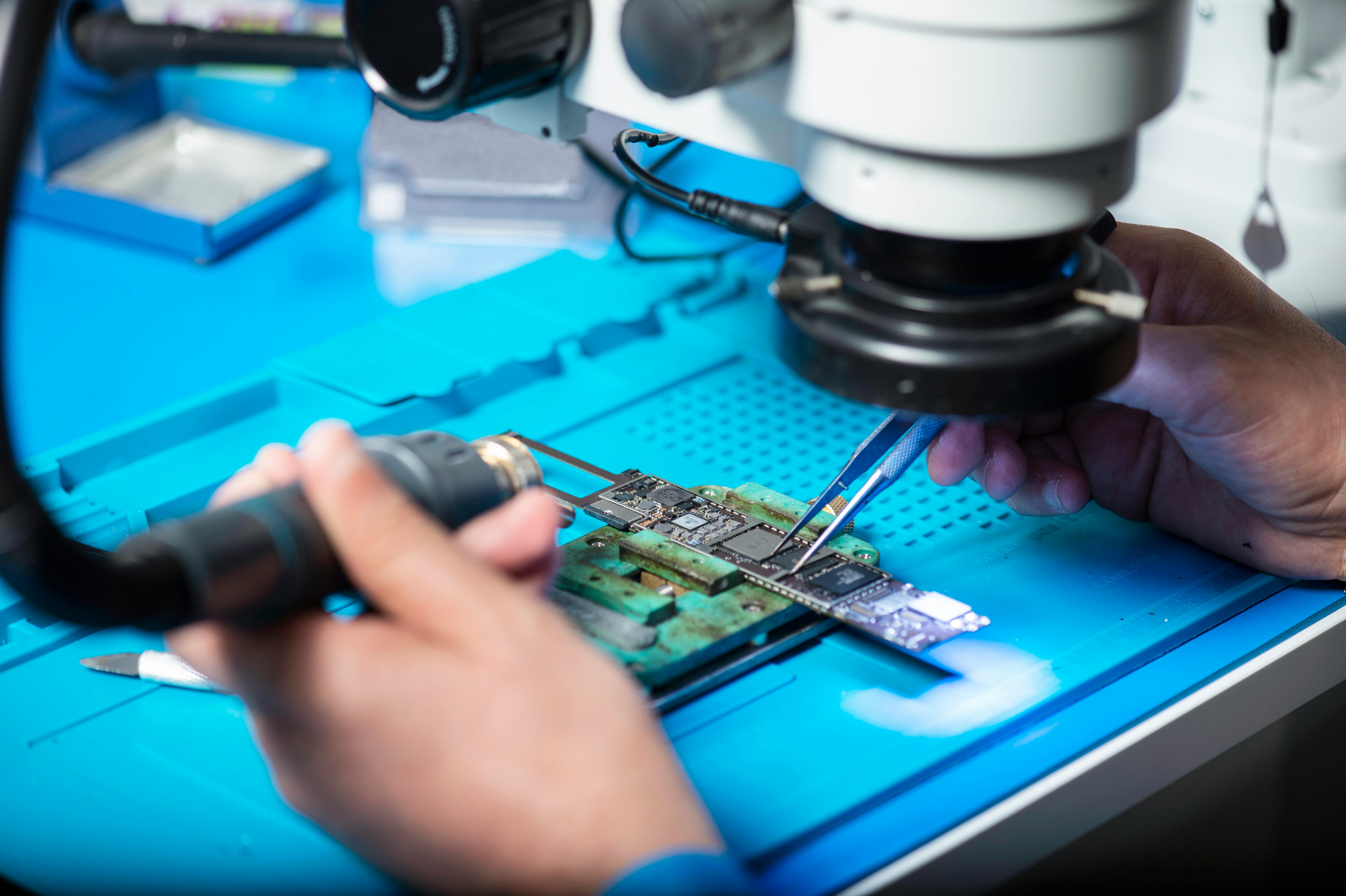
DeepMind and BioNTech, two leaders in artificial intelligence and biotechnology, are partnering to revolutionize scientific research through the development of AI-powered lab assistants. These advanced AI systems aim to assist researchers in planning experiments, predicting outcomes, and analyzing complex data. As scientific research becomes increasingly data-driven, the need for tools that can process vast amounts of information and optimize experimental designs has grown, making this collaboration a significant step toward more efficient and accurate research processes.
The Role of AI in Scientific Research
AI has already made significant inroads into various industries, from finance to healthcare, and is now becoming an essential tool in scientific research. In fields such as biotechnology, pharmaceuticals, and molecular biology, the complexity of data and the need for precise experimentation have outpaced traditional research methods. AI's ability to analyze large datasets, identify patterns, and make predictions offers a powerful solution to these challenges.
AI lab assistants are designed to tackle tasks that have traditionally required significant human input, such as designing experiments, selecting research parameters, and predicting the likely outcomes of studies. These systems aim to reduce human error, optimize time, and allow scientists to focus on creative problem-solving rather than manual data processing.
Capabilities of the AI Lab Assistants
The AI lab assistants being developed by DeepMind and BioNTech bring a range of sophisticated capabilities to the research environment. These systems can assist in designing complex experiments by analyzing previous research and suggesting optimal experimental setups. For example, in drug development, the AI assistant could recommend which chemical compounds to test or identify potential drug interactions, improving the efficiency of drug discovery.
Additionally, AI lab assistants are capable of predicting outcomes based on historical data, reducing the trial-and-error nature of traditional research. In areas like molecular biology, this could involve predicting how genetic modifications will affect a biological system, allowing scientists to prioritize the most promising experiments.
The AI systems also provide real-time analysis, offering researchers instant feedback on experimental results, which helps in refining approaches and adjusting parameters as needed. This level of adaptability and intelligence makes AI an invaluable tool for accelerating research timelines.
Impact on Experimentation and Research Efficiency
One of the most significant benefits of AI lab assistants is their potential to vastly improve research efficiency. By automating repetitive tasks such as data analysis and experimental design, these AI systems can save researchers both time and resources. This is particularly valuable in time-sensitive fields like pharmaceuticals, where the speed of drug discovery and development can directly impact patient outcomes.
AI-powered lab assistants can also help reduce costs associated with research. By optimizing experiments and reducing the number of failed tests, research organizations can allocate resources more effectively. In drug development, for example, AI could streamline the clinical trial process by better predicting which drug candidates are likely to succeed, potentially saving billions in development costs.
Early case studies in AI’s application to scientific research have already shown promising results, with some projects reporting reduced timelines for discovery and increased accuracy in experimental outcomes. As AI lab assistants become more widely adopted, these benefits could extend across various scientific fields.
Applications in Biotechnology and Drug Development
The biotechnology and drug development sectors stand to gain significantly from AI-powered lab assistants. In drug discovery, these AI systems can analyze vast chemical libraries to identify promising candidates for new therapies. They can also predict how drugs will interact with biological systems, speeding up the preclinical testing phase and improving the accuracy of predictions.
BioNTech, already known for its pioneering work in mRNA vaccine development, is leveraging AI to further its research into immunotherapies and other advanced treatments. AI assistants can help researchers design more effective vaccines by simulating immune responses, allowing for faster and more targeted vaccine development. This could prove especially valuable in the fight against rapidly evolving diseases.
In addition to drug discovery, AI lab assistants are poised to make significant contributions to areas like gene editing, where the precision of AI algorithms can help optimize genetic modifications, leading to more effective therapies for genetic disorders.
Challenges and Limitations
While the potential of AI lab assistants is substantial, there are challenges to integrating them into scientific research. One significant hurdle is data quality. AI systems rely on vast amounts of data to make accurate predictions, and if the data used is incomplete or biased, the AI’s output may be flawed. Ensuring high-quality, representative datasets is critical for the success of AI-powered research tools.
Another challenge is the transparency of AI systems, often referred to as the "black box" problem. Researchers need to understand how AI arrives at certain conclusions to fully trust and utilize its recommendations. Developing AI systems that are explainable and transparent will be essential to gaining wider acceptance in the scientific community.
Additionally, while AI can greatly assist in planning and predicting outcomes, human oversight remains crucial. AI systems are tools, not replacements for researchers, and the ultimate success of these systems will depend on how effectively humans and machines can collaborate in the lab.
The Future of AI in Scientific Research
The collaboration between DeepMind and BioNTech represents just the beginning of what AI lab assistants can achieve in scientific research. As these systems evolve, they are likely to become even more integrated into the research process, with broader applications in fields such as materials science, environmental research, and personalized medicine.
For example, AI could help design new materials with specific properties by simulating molecular structures, or it could assist in environmental research by predicting the effects of climate change on ecosystems. In personalized medicine, AI could be used to tailor treatments to individual patients based on their genetic makeup, improving outcomes and reducing side effects.
The success of the DeepMind and BioNTech partnership could also set a precedent for other companies and research institutions to follow, accelerating the adoption of AI tools in labs around the world.
Conclusion
AI-powered lab assistants, as developed by DeepMind and BioNTech, have the potential to transform scientific research by improving efficiency, accuracy, and speed. These systems can assist researchers in planning complex experiments, predicting outcomes, and analyzing data, making them invaluable tools for modern science. While challenges remain, such as data quality and AI transparency, the benefits of integrating AI into the lab are clear.
As AI continues to evolve, its role in scientific discovery will only grow, offering new possibilities for breakthroughs in fields ranging from drug development to environmental science. DeepMind and BioNTech are leading the way in this transformation, demonstrating how AI and human expertise can work together to push the boundaries of what’s possible in scientific research.
Author: Ricardo Goulart
From Chip War To Cloud War: The Next Frontier In Global Tech Competition
The global chip war, characterized by intense competition among nations and corporations for supremacy in semiconductor ... Read more
The High Stakes Of Tech Regulation: Security Risks And Market Dynamics
The influence of tech giants in the global economy continues to grow, raising crucial questions about how to balance sec... Read more
The Tyranny Of Instagram Interiors: Why It's Time To Break Free From Algorithm-Driven Aesthetics
Instagram has become a dominant force in shaping interior design trends, offering a seemingly endless stream of inspirat... Read more
The Data Crunch In AI: Strategies For Sustainability
Exploring solutions to the imminent exhaustion of internet data for AI training.As the artificial intelligence (AI) indu... Read more
Google Abandons Four-Year Effort To Remove Cookies From Chrome Browser
After four years of dedicated effort, Google has decided to abandon its plan to remove third-party cookies from its Chro... Read more
LinkedIn Embraces AI And Gamification To Drive User Engagement And Revenue
In an effort to tackle slowing revenue growth and enhance user engagement, LinkedIn is turning to artificial intelligenc... Read more