AI Distillation: The Race To Build Smaller, Cheaper, And More Powerful Models
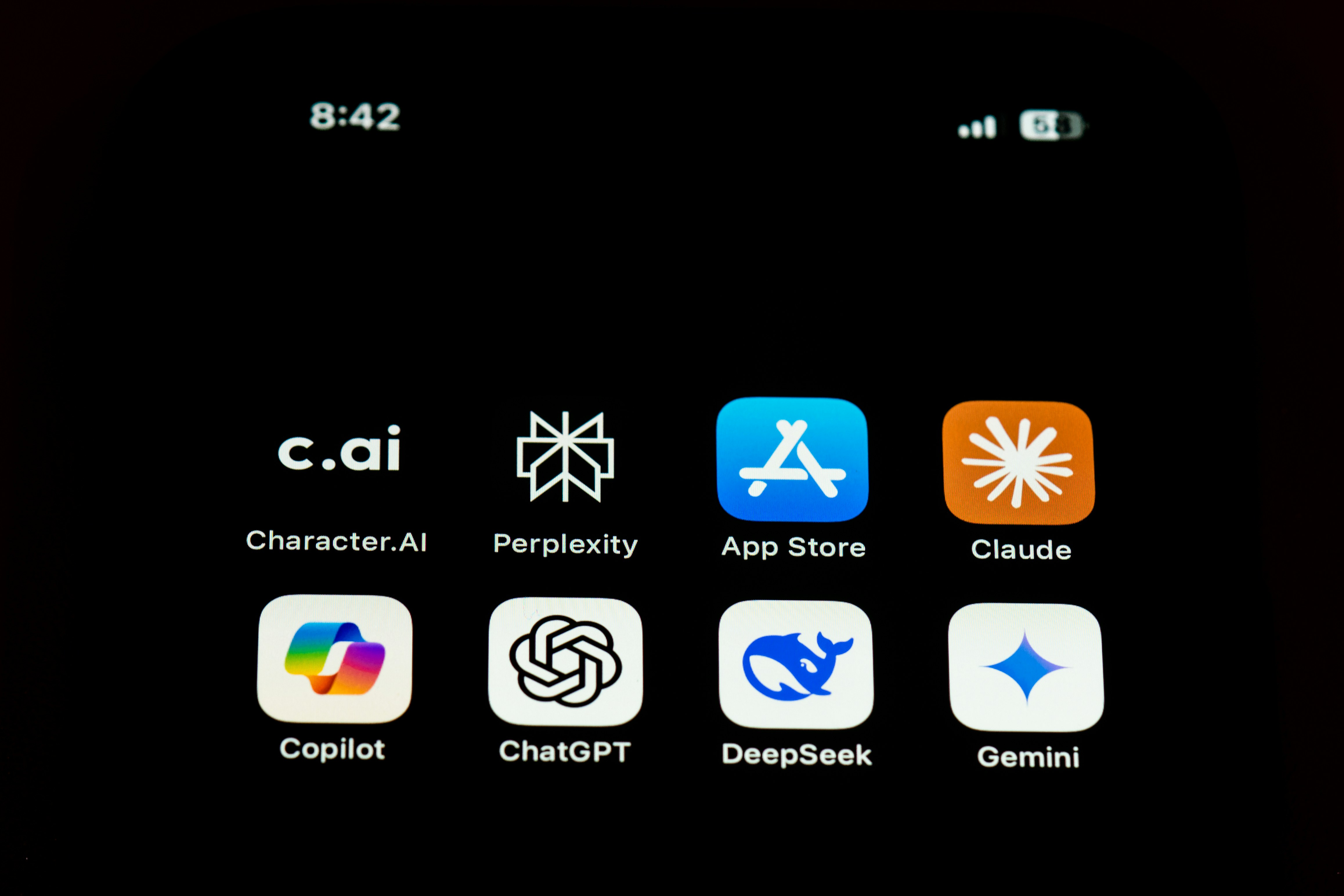
The artificial intelligence (AI) industry is engaged in an intense competition to build increasingly powerful models while reducing costs and computational demands. As AI applications expand, companies must find ways to optimize performance without requiring massive amounts of energy and expensive hardware.
One of the most promising techniques in this race is AI model distillation, a process that allows developers to create smaller models that retain the capabilities of their larger counterparts. Companies like DeepSeek are leveraging this approach to build efficient models based on the technology of competitors, such as Meta’s open-source AI models. But does distillation truly offer a path to cheaper, more accessible AI, or are there trade-offs that limit its effectiveness?
Understanding AI Model Distillation
What is Model Distillation?
Model distillation is an AI optimization technique in which a smaller “student” model is trained to replicate the performance of a larger “teacher” model. The student model learns by mimicking the teacher’s outputs, often through a process of transferring knowledge while filtering out unnecessary complexities.
This technique allows AI developers to compress large models into smaller versions that can still perform well in tasks such as natural language processing, image recognition, and decision-making.
Advantages of Distillation
- Cost Reduction – Smaller models require less computational power, reducing training and operational costs.
- Energy Efficiency – By requiring fewer resources, distilled models consume less electricity, making AI more sustainable.
- Faster Deployment – Smaller AI models can be deployed on less powerful hardware, including mobile devices and edge computing systems.
- Maintaining Performance – With proper optimization, a well-distilled model can achieve similar accuracy to a full-scale model while being much more efficient.
The Competitive Landscape of AI Distillation
Major Players in the AI Distillation Race
AI distillation has become a core focus for major AI companies, each developing their own approach:
- DeepSeek has gained attention for using distillation to create competitive AI models built on the foundation of open-source models from companies like Meta.
- Meta has released open-source models like Llama, providing a foundation that other companies can distill into smaller, optimized versions.
- Google and OpenAI are also exploring distillation to refine their language models, aiming to maintain performance while cutting costs.
The Push for Efficiency in AI Development
The demand for smaller, more efficient models is being driven by several factors:
- The rising costs of training large AI models, which require immense computing power.
- The need for AI democratization, allowing smaller businesses and researchers to access powerful AI without requiring massive infrastructure.
- The expansion of AI into mobile and edge computing, where processing power is limited, making efficiency crucial.
Companies that successfully implement distillation gain a competitive edge by offering cost-effective AI solutions without compromising performance.
Challenges and Limitations of AI Distillation
Performance Trade-offs
While distillation reduces model size, there can be a loss of complexity, leading to:
- Weaker reasoning capabilities in distilled models.
- Reduced generalization across a wide range of tasks compared to full-scale models.
- Possible errors in nuanced AI applications, such as those requiring deep contextual understanding.
Ethical and Intellectual Property Concerns
- Using competitor models as a foundation for distillation raises questions about intellectual property.
- Open-source vs. proprietary AI models – While some companies encourage collaboration, others worry that their models are being used unfairly.
- Data ownership and bias concerns – If a distilled model inherits biases from the teacher model, it could reinforce existing flaws in AI decision-making.
Scalability and Future Limitations
- Can distillation keep pace with the rapid growth of AI model sizes?
- Will new techniques emerge to overcome the inherent trade-offs?
- The industry needs to continue refining distillation methods to ensure long-term viability.
The Future of AI Model Distillation
The Role of Open-Source AI in Advancing Distillation
Open-source AI plays a crucial role in distillation by:
- Providing a foundation for companies to develop competitive AI models.
- Encouraging innovation through collaboration.
- Lowering the barrier for smaller firms to enter the AI market.
Potential for More Accessible AI
- Smaller AI models mean AI can be deployed on a wider range of devices, from smartphones to embedded systems.
- Businesses and researchers without access to high-end computing resources can still work with powerful AI tools.
- AI expansion into emerging markets, where computational infrastructure is often limited.
What’s Next for AI Distillation?
- New advancements in AI training techniques to further improve distillation outcomes.
- The impact of AI hardware developments (e.g., more efficient chips) on the demand for smaller models.
- Continued competition between AI giants, driving further innovation in cost-effective AI deployment.
Conclusion
AI distillation is proving to be a crucial tool in the race to build smaller, cheaper, and more powerful models. Companies like DeepSeek, Meta, and Google are leveraging this technique to create efficient AI that can operate with lower costs and on less powerful hardware.
However, while distillation offers many advantages, it is not a perfect solution. Performance trade-offs, ethical concerns, and the ever-growing complexity of AI models present ongoing challenges.
As AI continues to evolve, the success of distillation will depend on how well researchers refine the technique and integrate it with emerging technologies. One thing is clear: the push for smaller, more efficient AI is only just beginning.
Author: Ricardo Goulart
From Chip War To Cloud War: The Next Frontier In Global Tech Competition
The global chip war, characterized by intense competition among nations and corporations for supremacy in semiconductor ... Read more
The High Stakes Of Tech Regulation: Security Risks And Market Dynamics
The influence of tech giants in the global economy continues to grow, raising crucial questions about how to balance sec... Read more
The Tyranny Of Instagram Interiors: Why It's Time To Break Free From Algorithm-Driven Aesthetics
Instagram has become a dominant force in shaping interior design trends, offering a seemingly endless stream of inspirat... Read more
The Data Crunch In AI: Strategies For Sustainability
Exploring solutions to the imminent exhaustion of internet data for AI training.As the artificial intelligence (AI) indu... Read more
Google Abandons Four-Year Effort To Remove Cookies From Chrome Browser
After four years of dedicated effort, Google has decided to abandon its plan to remove third-party cookies from its Chro... Read more
LinkedIn Embraces AI And Gamification To Drive User Engagement And Revenue
In an effort to tackle slowing revenue growth and enhance user engagement, LinkedIn is turning to artificial intelligenc... Read more