What's Going On With Eos, Nvidia's Incredible Shrinking Supercomputer?
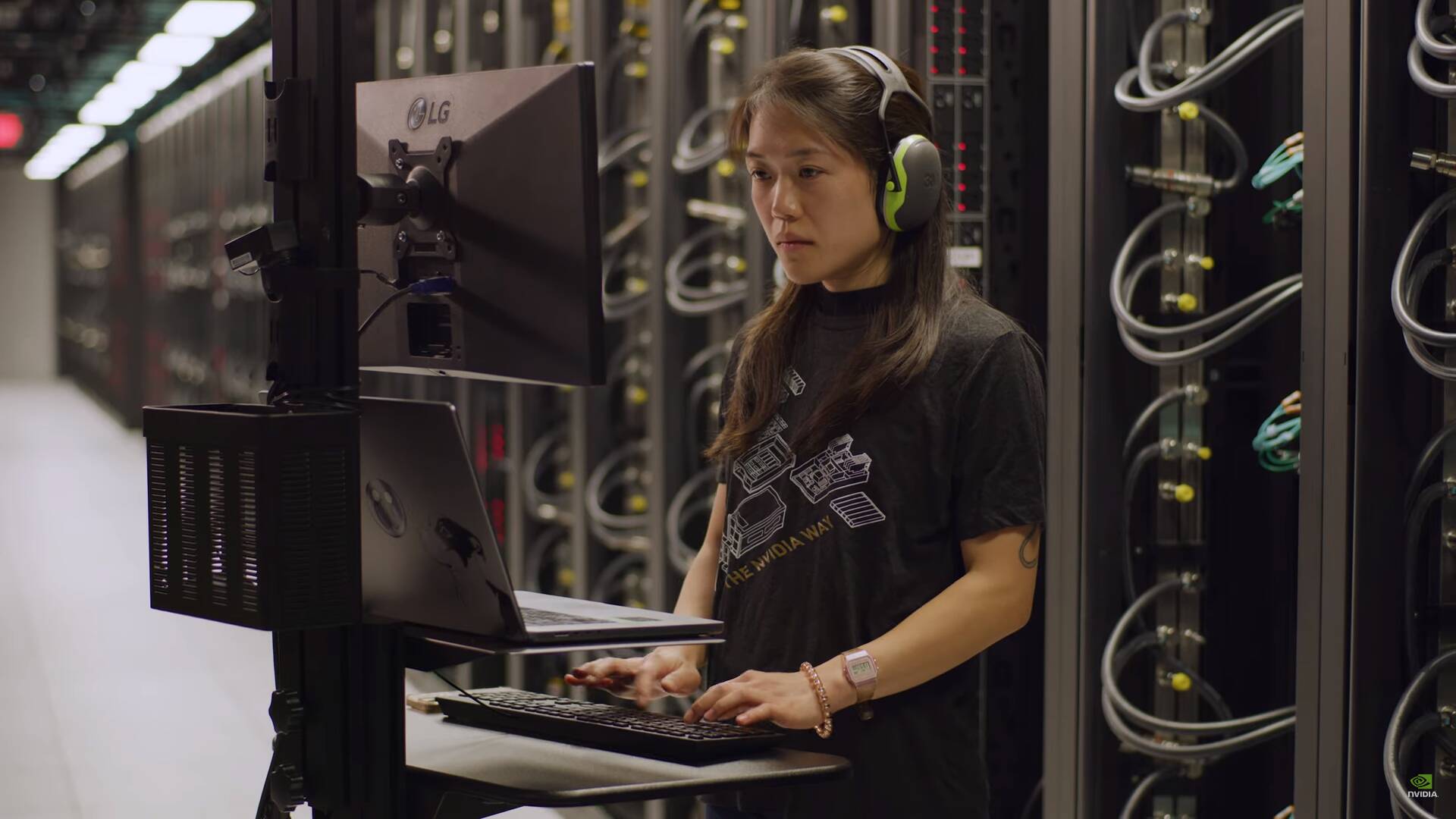
Updated Nvidia can't seem to make up its mind just how big its Eos supercomputer is.
In a blog post this month re-revealing the ninth most-powerful publicly known supercomputer from last fall's Top500 ranking, the GPU slinger said Eos was built using 576 DGX H100 systems totaling 4,608 GPUs. That's about what we expected when the computer was announced.
While impressive in its own right, that's less than half the number of GPUs Nvidia claimed the system had in November when it took to the web to talk up the machine's performance in a variety of MLPerf AI training benchmarks.
Back then, the Eos super apparently had a complement of 10,752 H100 GPUs, which would have spanned 1,344 DGX systems. With nearly 4 petaFLOPS of sparse FP8 performance per GPU, that supercomputer would have been capable of 42.5 exaFLOPS of peak AI compute. Compare that to the 18.4 AI exaFLOPS Nvidia says the system is capable of outputting today, and Eos appears to have lost some muscle tone.
And if you're not familiar with the term AI exaFLOPS, it's a metric commonly used to describe floating-point performance at a lower precision than you'd typically see in double-precision HPC benchmarks, such as LINPACK. In this case, Nvidia is arriving at these figures using sparse 8-bit floating-point math, but another vendor, like Cerebras, might calculate AI FLOPS using FP16 or BF16.
So where did the other 60 percent of the Eos system go? We put this question to Nvidia and were told "the supercomputer used for MLPerf LLM training with 10,752 H100 GPUs is a different system built with the same DGX SuperPOD architecture."
"The system ranked number nine on the 2023 TOP500 list is the 4,608 GPU Eos system featured in today's blog post and video," the spokesperson added.
Except that doesn't appear to be true either. Eos's Top500 score of 121 petaFLOPS of FP64 out of an estimated peak of 188.65 is too low. The latter should be somewhere between the 275 petaFLOPS originally claimed and the 308 petaFLOPS of FP64 that Nvidia's spec sheet says 4,608 H100s should actually net you.
So while Nvidia hasn't admitted exactly how many GPUs were used, based on these performance figures we can estimate the November run was made using somewhere between 2,816 and 3,161 GPUs.
Nvidia's decision to put forward a smaller version of the system on last fall's Top500 ranking, when it had already demonstrated a much larger Eos cluster, strikes us as odd.
With more than ten thousand H100s on board, the larger Eos config would have boasted 720 petaFLOPS of peak double-precision performance. Granted, real-world performance would have been a fair bit lower.
- Oxide reimagines private cloud as... a 3,000-pound blade server?
- Someone had to say it: Scientists propose AI apocalypse kill switches
- Microsoft says it'll throw €3.2B at AI ops in Germany
- Upstart retrofits an Nvidia GH200 server into a €47,500 workstation
We asked Nvidia for clarification on these discrepancies and were told that the timeline didn't permit for a Top500 run on the larger system. Why? They didn't say. "Our teams are racing towards GTC and are not able to provide more details on last year's TOP500 submission at this time," a spokesperson told The Register.
Having said that, Nvidia wouldn't be the only one that couldn't get a full run of their machine done in time. Argonne National Laboratory's Aurora supercomputer, the flagship for Intel's Xeon and GPU Max families, was only able to manage a partial run too. This suggests we may catch a glimpse of an even more powerful Eos system on this spring's Top500.
In any case, Eos's shapeshifting does highlight one of the conveniences associated with Nvidia's modular DGX SuperPOD architecture. It can be scaled out and broken into chunks depending on what it's needed for.
Each SuperPod is made up of what Nvidia calls scalable units (SU) containing 32 DGX H100 nodes containing eight GPUs connected via its 400Gb/s Quantum-2 InfiniBand network. Additional SUs can be added to scale the system to support larger workloads. Officially, Nvidia supports up to four SUs per pod, but the silicon goliath notes that larger configurations are possible, which is clearly the case with Eos.
As for how big Eos really is, it appears the answer to that depends entirely on how big Nvidia wants it to be at any given moment. ®
Updated to add on February 21
Spokespeople for Nvidia have been in touch hoping to further clarify this Eos saga.
From what we can tell, in 2022, Nvidia said it was building an Eos supercomputer with 4,608 H100 accelerators. By November last year, Nv said the machine had swelled to 10,752 GPUs. Last week, the silicon giant brought up the super again, and it's suddenly back down to 4,608 GPUs and it turns out this was the hardware used to submit a speed-run to the Top500.
Now it's clear Nvidia used a portion of the 4K Eos, specifically 3,328 H100 accelerators, for that Top500 run. It didn't enter the full 10K monster — which is confusingly an entirely different machine by the same name, Eos — nor the full 4K Eos because it needed the remaining GPUs for more important internal work, we've learned.
Also, Microsoft had built a supercomputer described as a physical twin of the larger Eos machine – presumably this beast – and submitted a benchmark run on it to the Top500, so Nvidia felt that base was covered one way or another.
What with all the confusion over the specs, we speculated Nvidia may have run into trouble with stability on the full cluster. Nv's reps have since stressed to us that it would be wrong to assume there was some kind of instability limiting the size of the machine.
Finally, Nvidia said it has updated the above-linked blog post with more details to potentially straighten out this whole affair. We'll let you be the judge.
Want more analysis? Getting your hands on a H100 GPU is probably the most difficult thing in the world right now – even for Nvidia itself.
From Chip War To Cloud War: The Next Frontier In Global Tech Competition
The global chip war, characterized by intense competition among nations and corporations for supremacy in semiconductor ... Read more
The High Stakes Of Tech Regulation: Security Risks And Market Dynamics
The influence of tech giants in the global economy continues to grow, raising crucial questions about how to balance sec... Read more
The Tyranny Of Instagram Interiors: Why It's Time To Break Free From Algorithm-Driven Aesthetics
Instagram has become a dominant force in shaping interior design trends, offering a seemingly endless stream of inspirat... Read more
The Data Crunch In AI: Strategies For Sustainability
Exploring solutions to the imminent exhaustion of internet data for AI training.As the artificial intelligence (AI) indu... Read more
Google Abandons Four-Year Effort To Remove Cookies From Chrome Browser
After four years of dedicated effort, Google has decided to abandon its plan to remove third-party cookies from its Chro... Read more
LinkedIn Embraces AI And Gamification To Drive User Engagement And Revenue
In an effort to tackle slowing revenue growth and enhance user engagement, LinkedIn is turning to artificial intelligenc... Read more