Future-Proofing AI: Developing Robust Evaluation Frameworks For Rapidly Evolving Models
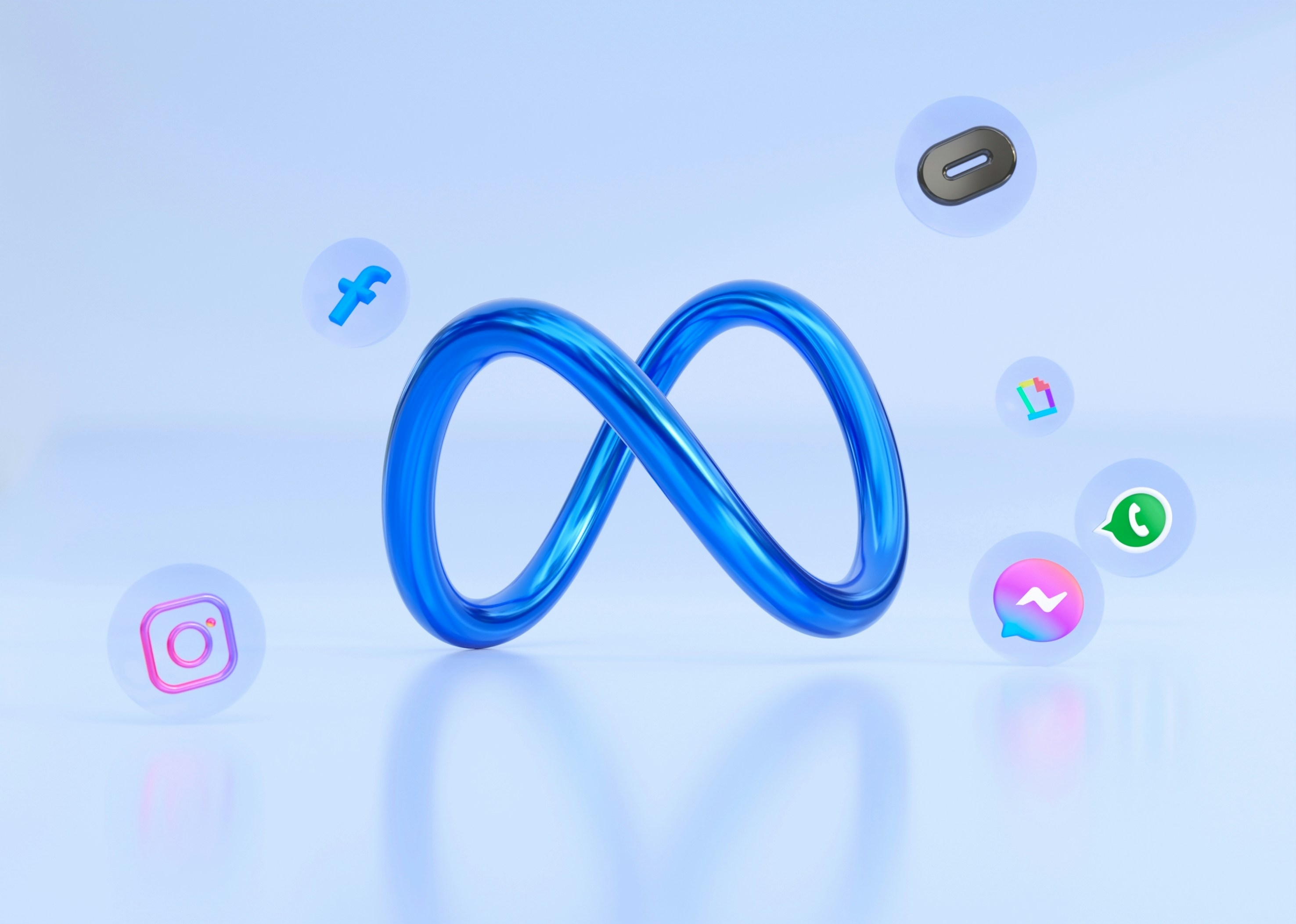
As artificial intelligence (AI) continues to evolve at an unprecedented pace, particularly with large language models (LLMs), the need for adaptable and robust evaluation frameworks is more pressing than ever. Traditional benchmarks and metrics that served well in earlier stages of AI development are quickly becoming outdated. In this article, we will explore the limitations of current evaluation methods, discuss the challenges of creating new frameworks, and present strategies for future-proofing AI testing to better handle advanced and continually advancing technologies.
Limitations of Current Evaluation Frameworks
Outdated Benchmarks and Metrics
Many of today’s traditional benchmarks fail to capture the sophisticated capabilities of modern AI models. Metrics designed to measure basic tasks like translation or sentence completion don’t fully address the complex functions of today’s AI systems, which include advanced reasoning, contextual understanding, and ethical decision-making. Consequently, existing benchmarks often fall short in evaluating how accurately these models perform in nuanced, real-world applications.
Inability to Capture Emergent Capabilities
With advances in AI, models now exhibit emergent behaviors—unexpected abilities and responses that were not directly programmed. These capabilities, which can include creative problem-solving, ethical reasoning, and even cultural sensitivity, are not adequately measured by current benchmarks. Evaluation frameworks, therefore, must evolve to assess such emergent capabilities, as they play a significant role in determining the model’s real-world functionality and adaptability.
Risks of Over-Reliance on Static Benchmarks
Static benchmarks can encourage “benchmark gaming,” where developers optimize models to perform well on specific tests without genuinely advancing their broader functionality. This focus can result in AI systems that perform exceptionally well in controlled settings but struggle to meet practical requirements in dynamic environments. Over-reliance on these benchmarks thus risks creating models that lack versatility and generalizability.
Key Challenges in Developing New Frameworks
Balancing Complexity with Practicality
Creating comprehensive evaluation frameworks that effectively measure AI capabilities is no small feat. These frameworks need to be intricate enough to assess complex model behaviors while remaining practical and interpretable for researchers and developers. Achieving this balance is essential to ensure that testing frameworks can be widely used and understood without becoming overly cumbersome or opaque.
Handling Diverse Model Architectures and Purposes
As AI model architectures become increasingly varied, with models specialized in vision, language, and multimodal tasks, evaluation frameworks must account for these differences. A universal set of benchmarks may not be feasible, as models have distinct purposes and strengths. Developing adaptable benchmarks that address diverse architectures and applications will be essential to fairly and accurately assess a wide range of models.
Ensuring Ethical and Safety Considerations
Another significant challenge is incorporating ethical and safety metrics into evaluation frameworks. As AI systems are integrated into sensitive areas like healthcare, criminal justice, and education, assessing bias, fairness, and safety becomes crucial. These considerations ensure that AI models align with human values and avoid harmful or unethical outcomes. Thus, future evaluation frameworks must go beyond performance metrics to consider ethical dimensions.
Future-Proofing Strategies for Robust Evaluation
Dynamic and Adaptive Benchmarking Systems
To future-proof AI evaluation, one promising approach is to create adaptable benchmarks that can evolve alongside model capabilities. Rather than relying on static metrics, dynamic benchmarking systems allow for ongoing updates as new capabilities or behaviors emerge. Modular testing can be incorporated, enabling researchers to add new assessments without needing to overhaul entire frameworks, making evaluation more responsive and up-to-date.
Real-World Performance and Human-AI Interaction Metrics
Testing AI in simulated real-world applications provides a more accurate measure of model performance and utility. By evaluating models based on how they perform in practical scenarios—such as in customer support interactions or creative content generation—researchers can better understand their real-world functionality. Metrics that assess human-AI interaction, including user experience and interpretability, also provide valuable insights into how effectively models support human decision-making and enhance productivity.
Multidimensional Metrics for Comprehensive Assessment
A multidimensional approach to evaluation integrates various types of metrics, such as linguistic accuracy, contextual relevance, ethical considerations, and task-specific performance. These dimensions allow for a more balanced and holistic assessment of AI models, ensuring that testing captures a range of critical capabilities. By evaluating multiple aspects simultaneously, these frameworks can provide a nuanced understanding of a model’s strengths, weaknesses, and areas for improvement.
Collaborative Efforts in the AI Community
Open-Source Benchmarking Initiatives
Open-source benchmarking initiatives have emerged as an effective way to promote transparency and encourage collaboration within the AI community. These initiatives allow researchers and developers to contribute to and refine evaluation frameworks, fostering a shared standard for testing advanced models. Open-source projects also facilitate innovation, as contributors from diverse backgrounds can bring unique perspectives to the evaluation process.
Industry and Academic Partnerships
Partnerships between industry and academia are essential for advancing AI evaluation. By pooling resources, expertise, and data, these collaborations can accelerate the development of robust benchmarks that meet the needs of both researchers and commercial entities. Examples include joint research projects and shared datasets that help establish standards for next-generation AI models, benefiting both the academic and business communities.
Involvement of Policy and Ethical Standards Organizations
Policy and ethical standards organizations play a crucial role in guiding the development of future-proof AI evaluation frameworks. These organizations provide guidelines to ensure that AI systems align with societal values, addressing critical issues like data privacy, bias, and transparency. Collaborating with such bodies can help the AI community develop testing standards that promote ethical and responsible AI use, supporting compliance with future regulations.
Looking Ahead: The Role of Adaptable Frameworks in Future AI
Anticipating Continuous Model Evolution
As AI capabilities continue to expand, the need for adaptable evaluation frameworks will only grow. AI researchers must anticipate that new types of models will emerge, bringing unforeseen capabilities and behaviors. By designing evaluation systems that can evolve over time, the AI community can stay prepared for future developments and ensure that testing standards remain relevant.
The Need for Flexibility in Ethical and Regulatory Compliance
Adaptable evaluation frameworks will be crucial for ensuring compliance with evolving AI regulations and ethical guidelines. As governments around the world consider new policies for AI safety, transparency, and fairness, flexible frameworks can help the AI community stay aligned with regulatory standards. This flexibility will also support developers in building models that meet societal expectations and legal requirements.
Long-Term Impact on AI Research and Development
Robust, adaptable evaluation systems can have a positive impact on AI research and development. Future-proofing evaluation frameworks ensures that AI models are built and assessed with a focus on real-world applicability, ethical responsibility, and safety. By fostering innovation within responsible boundaries, these systems encourage the creation of AI technologies that are beneficial, reliable, and aligned with human interests.
Conclusion
The rapid evolution of AI technology calls for equally dynamic and adaptable evaluation frameworks. Traditional benchmarks are no longer sufficient to assess the capabilities of advanced models, and future-proofing our testing methods has become essential. By addressing current limitations, meeting development challenges, and leveraging collaboration, the AI community can build robust evaluation frameworks that promote responsible, ethical, and innovative advancements in AI. As AI continues to transform society, these frameworks will play a pivotal role in shaping a future where technology serves the greater good.
Author: Ricardo Goulart
From Chip War To Cloud War: The Next Frontier In Global Tech Competition
The global chip war, characterized by intense competition among nations and corporations for supremacy in semiconductor ... Read more
The High Stakes Of Tech Regulation: Security Risks And Market Dynamics
The influence of tech giants in the global economy continues to grow, raising crucial questions about how to balance sec... Read more
The Tyranny Of Instagram Interiors: Why It's Time To Break Free From Algorithm-Driven Aesthetics
Instagram has become a dominant force in shaping interior design trends, offering a seemingly endless stream of inspirat... Read more
The Data Crunch In AI: Strategies For Sustainability
Exploring solutions to the imminent exhaustion of internet data for AI training.As the artificial intelligence (AI) indu... Read more
Google Abandons Four-Year Effort To Remove Cookies From Chrome Browser
After four years of dedicated effort, Google has decided to abandon its plan to remove third-party cookies from its Chro... Read more
LinkedIn Embraces AI And Gamification To Drive User Engagement And Revenue
In an effort to tackle slowing revenue growth and enhance user engagement, LinkedIn is turning to artificial intelligenc... Read more