Exploring The Neural Networks Of AI: How Baby-Like Learning Enhances Machine Understanding
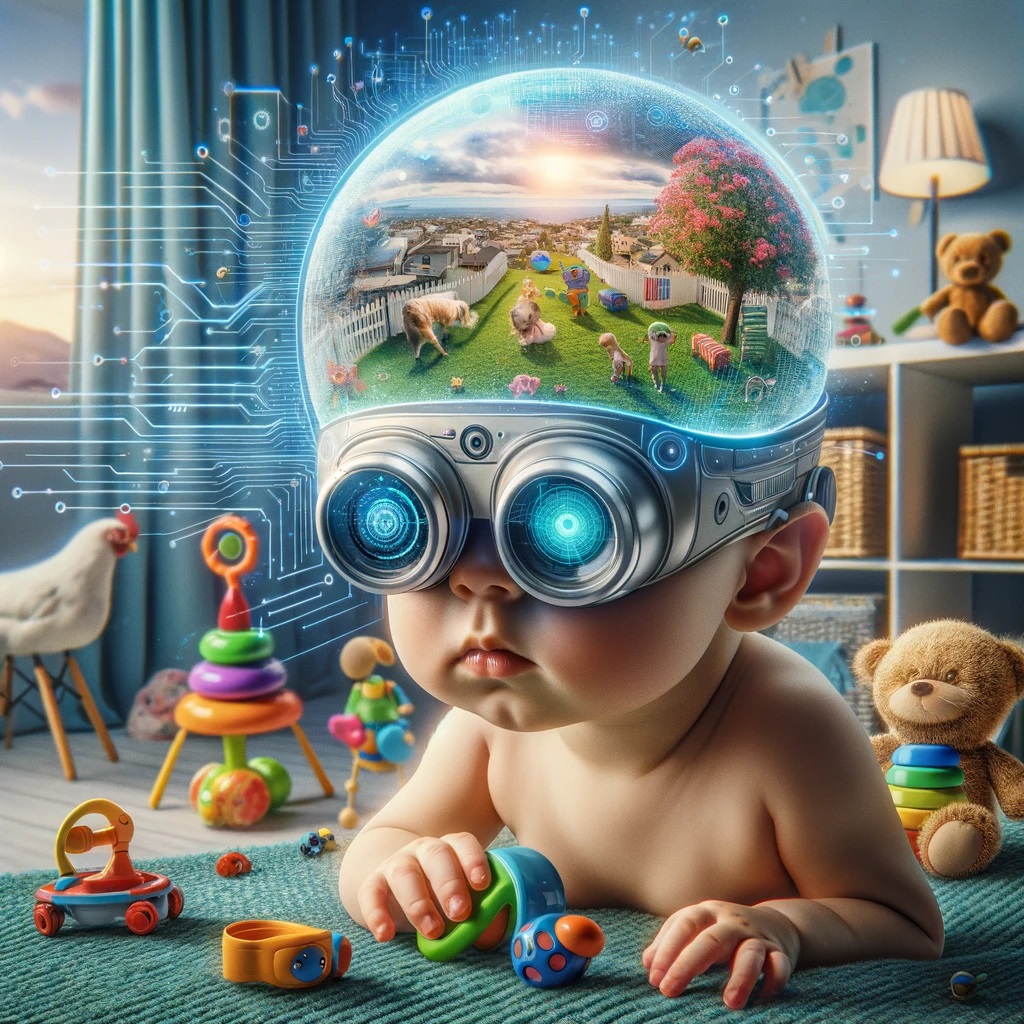
In an era dominated by artificial intelligence (AI) advancements, the quest for machines that understand and interact with the human world in more intuitive ways has led scientists down a novel path. Traditionally, AI models such as GPT-4 have been trained on vast databases of text, amassing language skills through the analysis of millions of web pages. This method, while effective in creating highly knowledgeable AIs, lacks a fundamental component of human learning: experience.
A groundbreaking experiment by a team of scientists at New York University challenges the status quo, offering a glimpse into an AI's potential to learn language through the eyes of a baby. This innovative approach diverges from the digital immersion of AI in textual data, opting instead for a more organic learning process rooted in the visual and auditory experiences of a toddler named Sam. Between six and 25 months old, Sam wore a head-mounted camera for an hour a week, capturing his interactions with the world—playing with toys, spending days at the park, and mingling with his pet cats. The recorded data, a cacophony of colors, movements, and sounds, was then fed into an AI model. This model was designed to associate images with words, mimicking the way a child learns to link objects with their names.
The results of this experiment were both promising and surprising. The AI demonstrated a remarkable ability to identify objects and their corresponding words with a 62% success rate, significantly surpassing the mere 25% chance level. Even more intriguing was the AI's capacity to recognize chairs and balls that Sam had never encountered during the experiment, suggesting an ability to generalize its learned knowledge to new situations. With at least 40 different words in its repertoire, the AI's achievements, though modest compared to a toddler's vocabulary, mark a significant step forward in machine learning.
This success story, however, opens up a broader conversation about the methodologies employed in AI development. The traditional approach, reliant on textual data, has undeniably propelled AI to new heights. Yet, it inherently lacks the messiness and unpredictability of real-world experiences that shape human cognition from infancy. The NYU experiment sheds light on an alternative pathway, one that mimics the human experience more closely, potentially paving the way for AI systems that understand the world in a more nuanced and adaptable manner.
Critics of the experiment raise valid concerns, questioning the scalability of such a method and its applicability beyond the realm of tangible, visible objects. Learning abstract nouns or verbs, they argue, might prove a far more challenging task for AI models trained in this experiential manner. Furthermore, the debate continues on how closely these AI learning processes can truly mimic human language acquisition, a complex interplay of innate capabilities and environmental stimuli.
The implications of the NYU team's work extend beyond the confines of academic discourse, offering a tantalizing glimpse into the future of AI development. By integrating experiential learning into AI training regimes, developers could usher in a new era of machines that not only comprehend but also perceive the world with a semblance of human intuition. Future research, expanding on the foundational work of the NYU experiment, is crucial. As AI continues to evolve, the quest for models that can navigate the complexity of human language and experience remains a compelling frontier, promising advancements that could redefine our interaction with technology.
In conclusion, the experiment conducted by the scientists at New York University represents a pivotal moment in the ongoing exploration of AI's potential. By stepping away from the digital confines of text-based learning and embracing the chaotic tapestry of human experience, this research offers a promising avenue for developing AI that understands the world in a way that more closely mirrors our own cognitive processes. As we stand on the brink of these potential advancements, the importance of innovative approaches in AI training cannot be overstated. The journey towards creating machines that learn like us, with all the unpredictability and richness that entails, is just beginning.
Author: Brett Hurll
From Chip War To Cloud War: The Next Frontier In Global Tech Competition
The global chip war, characterized by intense competition among nations and corporations for supremacy in semiconductor ... Read more
The High Stakes Of Tech Regulation: Security Risks And Market Dynamics
The influence of tech giants in the global economy continues to grow, raising crucial questions about how to balance sec... Read more
The Tyranny Of Instagram Interiors: Why It's Time To Break Free From Algorithm-Driven Aesthetics
Instagram has become a dominant force in shaping interior design trends, offering a seemingly endless stream of inspirat... Read more
The Data Crunch In AI: Strategies For Sustainability
Exploring solutions to the imminent exhaustion of internet data for AI training.As the artificial intelligence (AI) indu... Read more
Google Abandons Four-Year Effort To Remove Cookies From Chrome Browser
After four years of dedicated effort, Google has decided to abandon its plan to remove third-party cookies from its Chro... Read more
LinkedIn Embraces AI And Gamification To Drive User Engagement And Revenue
In an effort to tackle slowing revenue growth and enhance user engagement, LinkedIn is turning to artificial intelligenc... Read more