Developing AI Models Or Giant GPU Clusters? Uncle Sam Would Like A Word
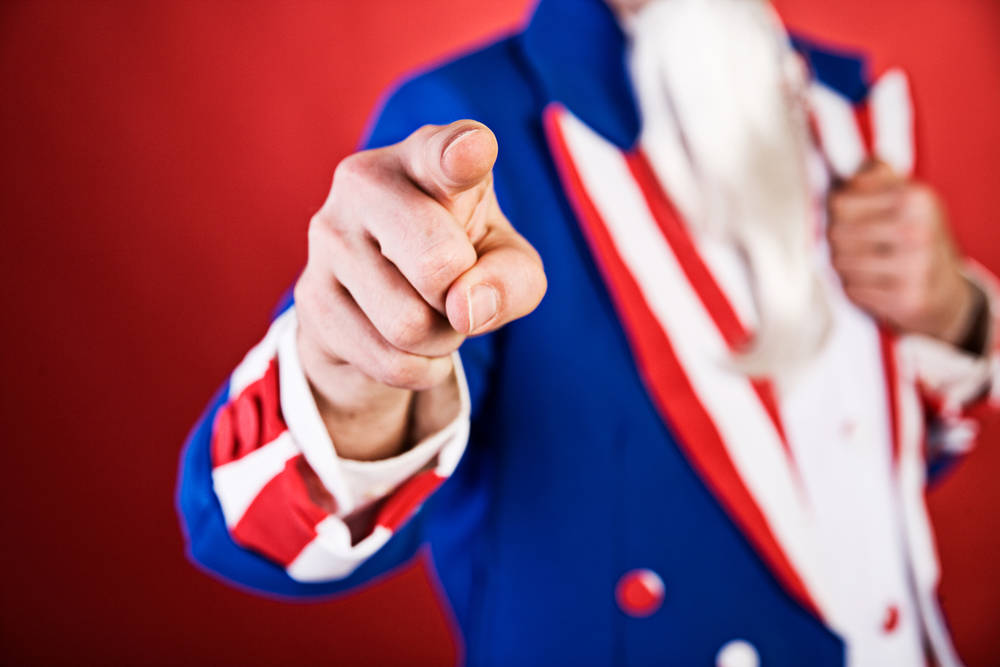
Analysis The White House wants to know who is deploying AI compute clusters and training large language models — but for now only the really, really, big ones.
In an executive order signed this week, US President Joe Biden laid out his agenda for ensuring the safe and productive development of AI technologies.
Among the directives was a requirement for operators of AI compute clusters and models exceeding certain thresholds to tell Uncle Sam what they run and where they run it. A closer look at the details of that requirement suggests only the very largest ML companies and infrastructure providers will be compelled to detail their activities.
The administration wants to know about the development of potential dual-use foundation models, what security measures are being made to protect them, and what steps they're using to prevent misuse. Dual use meaning the neural networks can be used in peaceful civilian and non-peaceful military applications.
The White House also wants to know which companies possess, plan to own, or are in the process of building large scale AI clusters, plus the scale of the deployed compute power and location of facilities.
A look at the figures
So far the White House has only set interim thresholds that trigger reporting obligations.
One requires reporting of any model trained using more than 1026 integer or floating point operations total, or more than 1023 floating point operations for biological sequence data.
The second sets a threshold for compute clusters located in a single datacenter and networked at more than 100Gb/s. Facilities exceeding 1020 FLOPS of AI training capacity in that second case will be subject to reporting rules.
That 1020 FLOPS figure translates to 100 exaFLOPS, which is a lot for one datacenter. Meanwhile the 1026 figure is the cumulative number of operations used to train a model over a period of time and would be equivalent to a total of 100 million quintillion floating point operations.
Researchers at University of California, Berkeley estimate OpenAI's GPT-3 required about 3.1 x 1023 floating-point operations of compute to train the full 175 billion parameter model.
That’s well below the White House’s reporting threshold for a single model even though GPTs are just the sort of AI the administration professes to worry about. GPT-4, OpenAI's more advanced model, may also fall under the requirements.
"The common consensus seems to be that very few entities are going to be subject to it," Gartner analyst Lydia Clougherty Jones told The Register.
"When you're making a category, you do you have a sense of how many may fall into a category, and sometimes they're so broad that it's not even a category at all, it's almost everybody. This is the opposite of that."
This is, interestingly enough, at a time when OpenAI and others are being accused of encouraging tough regulations on powerful neural networks, out of a purported fear of them being dangerous to use, so that entrants to the industry are locked out. The big players in that case will have first mover advantage and the resources to meet the regulations, whereas smaller orgs will not.
Google Brain cofounder and AI guru Andrew Ng has been pretty clear with his views, that Big Tech is playing up ML risks to make life difficult for smaller rivals.
- Snowflake puts LLMs in the hands of SQL and Python coders
- UK bets on Intel CPUs and GPUs, Dell boxen, OpenStack for Dawn supercomputer
- UK govt finds £225M for Isambard-AI supercomputer powered by Nvidia
- Desperately seeking GPUs? AWS will let you reserve instances in advance – no refunds
By our estimate, individual models that meet the administration’s reporting threshold would employ a cluster of 10,000 Nvidia H100s running at their lowest precision with sparsity for about a month. However, many popular large language models, such as GPT-3, were trained at higher precision, which changes the math a bit. Using FP32, that same cluster would need to be run for 7.5 months to reach that limit.
The reporting requirement for AI datacenters is just as eyebrow raising, working out to 100 exaFLOPs. Note that neither rule addresses whether those limits are for FP8 calculations or FP64. As we've previously discussed 1 exaFLOPS at FP64 isn't the same as an 1 exaFLOPS at FP32 or FP8. Context matters.
Going back to the H100, you'd need a facility with about 25,000 of the Nvidia GPUs — each good for 3,958 teraFLOPS of sparse FP8 performance, to meet the reporting requirement. However, if you've deployed something like AMD's Instinct MI250X, which doesn't support FP8, you'd need 261,097 GPUs before the Biden administration wants you to fill in its reporting paperwork.
The Register is aware of H100 deployments at that scale. GPU-centric cloud operator CoreWeave has deployed about 22,000 H100s. AI infrastructure startup Voltage Park plans to deploy 24,000 H100s. However neither outfit puts all its GPUs in a single datacenter, so might not exceed the reporting threshold.
More precise reporting requirements are on the way. The US Secretary of Commerce has been directed to work with the Secretaries of State, Defense, and Energy, as well as the Director of National Intelligence to define and regularly update reporting rules for what systems and models will need to be reported to the Government. That group has been given 90 days to deliver their first set of rules.
This is the Biden Administration effectively saying: "We want to mandate something today, but we need 90 days to figure out exactly what those technical conditions should be," Gartner’s Clougherty Jones said.
In any case, we expect the number of organizations that will have to report their model developments and AI infrastructure build outs to Uncle Sam under the interim rules will be very small. ®
Bootnote
Speaking of machine learning and regulations, OpenAI, Google DeepMind, Amazon, Microsoft, Anthropic, Mistral, and Meta on Thursday signed a non-binding agreement with the UK, America, Singapore, Australia, Canada, the EU, Japan, and others (not China). In that pact, the businesses promised to test their powerful ML models for national security and other risks before releasing them to the wider world.
It was inked during the AI Summit taking place in the UK this week.
From Chip War To Cloud War: The Next Frontier In Global Tech Competition
The global chip war, characterized by intense competition among nations and corporations for supremacy in semiconductor ... Read more
The High Stakes Of Tech Regulation: Security Risks And Market Dynamics
The influence of tech giants in the global economy continues to grow, raising crucial questions about how to balance sec... Read more
The Tyranny Of Instagram Interiors: Why It's Time To Break Free From Algorithm-Driven Aesthetics
Instagram has become a dominant force in shaping interior design trends, offering a seemingly endless stream of inspirat... Read more
The Data Crunch In AI: Strategies For Sustainability
Exploring solutions to the imminent exhaustion of internet data for AI training.As the artificial intelligence (AI) indu... Read more
Google Abandons Four-Year Effort To Remove Cookies From Chrome Browser
After four years of dedicated effort, Google has decided to abandon its plan to remove third-party cookies from its Chro... Read more
LinkedIn Embraces AI And Gamification To Drive User Engagement And Revenue
In an effort to tackle slowing revenue growth and enhance user engagement, LinkedIn is turning to artificial intelligenc... Read more