Machine Learning For Fraud Detection In Retail Banking
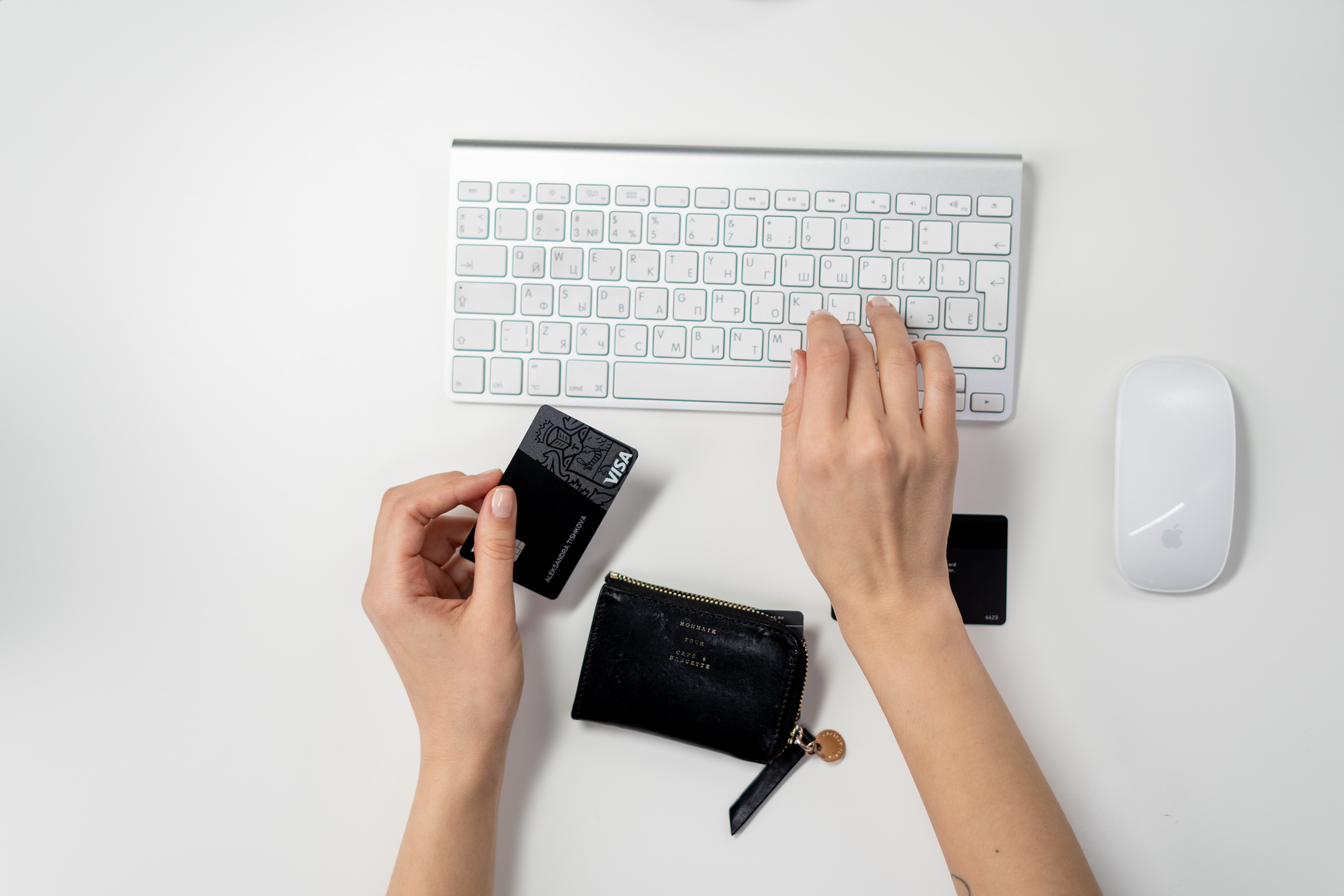
As digital landscapes transform, Machine Learning (ML) emerges as a pivotal technology, in particular for fraud detection in retail banking. Machine Learning, a subset of Artificial Intelligence, enables systems to learn, improve, and apply knowledge without explicit programming. Fraudulent activities, unfortunately, plague retail banking sectors worldwide, resulting in financial losses and undermined customer trust. The confluence of machine learning and fraud detection brings an innovative way to confront this challenge, enhancing security and efficiency.
Understanding Fraud in Retail Banking
'Fraud' in simplest terms means deceptive activities with financial gains as motive, varying from identity theft to credit card scams. Retail banking, as a significant interface between banks and customers, often witnesses frauds like credit card skimming or check fraud. Fraud not only inflicts substantial financial loss on banks but also diminishes customer confidence, impacting banks' reputation and customer loyalty. Consequently, the need for a robust system such as machine learning for fraud detection in retail banking is more pressing than ever.
Introduction of Machine Learning in Banking
Machine Learning is an intelligent system's capability to learn, adapt and improve from experience without being explicitly programmed. Banking sectors have started implementing machine learning algorithms in their operations to improve service efficiencies and customer experiences. For instance, they deploy ML for personalized customer service, risk management, predictive analysis, and most vitally, fraud detection.
Machine Learning in Fraud Detection
The integration of machine learning in fraud detection is transforming the retail banking landscape. Automated algorithms can scrutinize millions of transactions in real-time, pinpoint irregularities and stop fraudulent ones even before it happens. Technologies pivotal in ML implementation for fraud detection include anomaly detection methods, neural networks, and decision trees.
The benefits of using machine learning for fraud detection are multifold. By analyzing complex data, machine learning algorithms can identify suspicious patterns invisible to the human eye, enhancing the detection rate significantly. Such proactive fraud discovery mitigates potential financial losses, strengthens customer trust, and ensures seamless banking experience.
Future of Machine Learning in Fraud Detection
The progressive strides in machine learning technology enhance the prospects of fraud detection. Improved precision in identifying fraudulent transactions can stem from advancements in deep learning algorithms. However, challenges loom, such as managing data privacy concerns and the need for a delicate balance between false positive and detection accuracy.
Despite these challenges, the outlook for machine learning in fraud detection remains promising. With continuous upgrading of algorithms and improvement of data processing capability, machine learning’s effectiveness in curtailing fraudulent activities will steadily increase.
The intersection of machine learning and fraud detection makes for a powerfully effective tool in retail banking. The capability of ML to analyze vast amounts of data swiftly and efficiently helps banks stay ahead of sophisticated fraudulent activities. From enhancing customer trust to mitigating financial losses, machine learning’s role in fraud detection is increasingly crucial.
Looking ahead, the future of machine learning in fraud detection will likely be marked by constantly evolving algorithms and technology-driven advancements. It hints towards a dynamic retail banking landscape, continually adopting and upgrading to stay resilient against fraudsters' ingenious plots. The imperative and advantageous role of ML in fraud detection cannot be overstated, holding the key to secure and efficient banking.
Author: Brett Hurll
JPMorgan Deploys AI Chatbot To Revolutionize Research And Productivity
JPMorgan has deployed an AI-based research analyst chatbot to enhance productivity among its workforce, with approximate... Read more
Private Equity And Banks: The Complex Web Of Leverage
Private equity has emerged as a significant force in the global financial landscape, driving substantial growth and inve... Read more
Financial Watchdog Highlights Unresolved Vulnerabilities In Shadow Banking Sector
The world’s leading financial stability watchdog has issued a warning about the unresolved vulnerabilities within the ... Read more
JPMorgan And Small Caps Lead Market Rally: A Sign Of Economic Optimism
In a week marked by strong financial performance, JPMorgan Chase & Co. reported a 25% rise in profits, and US small-... Read more
Big Banks Vs. Regional Banks: The Battle For Market Share
The financial industry is a competitive landscape where big banks and regional banks vie for market share. Each type of ... Read more
The Evolution Of Philanthropic Advisory Services In Private Banks
The landscape of philanthropic advisory services provided by private banks has undergone a significant transformation. T... Read more